Unlocking the Potential of Data Annotation in Machine Learning
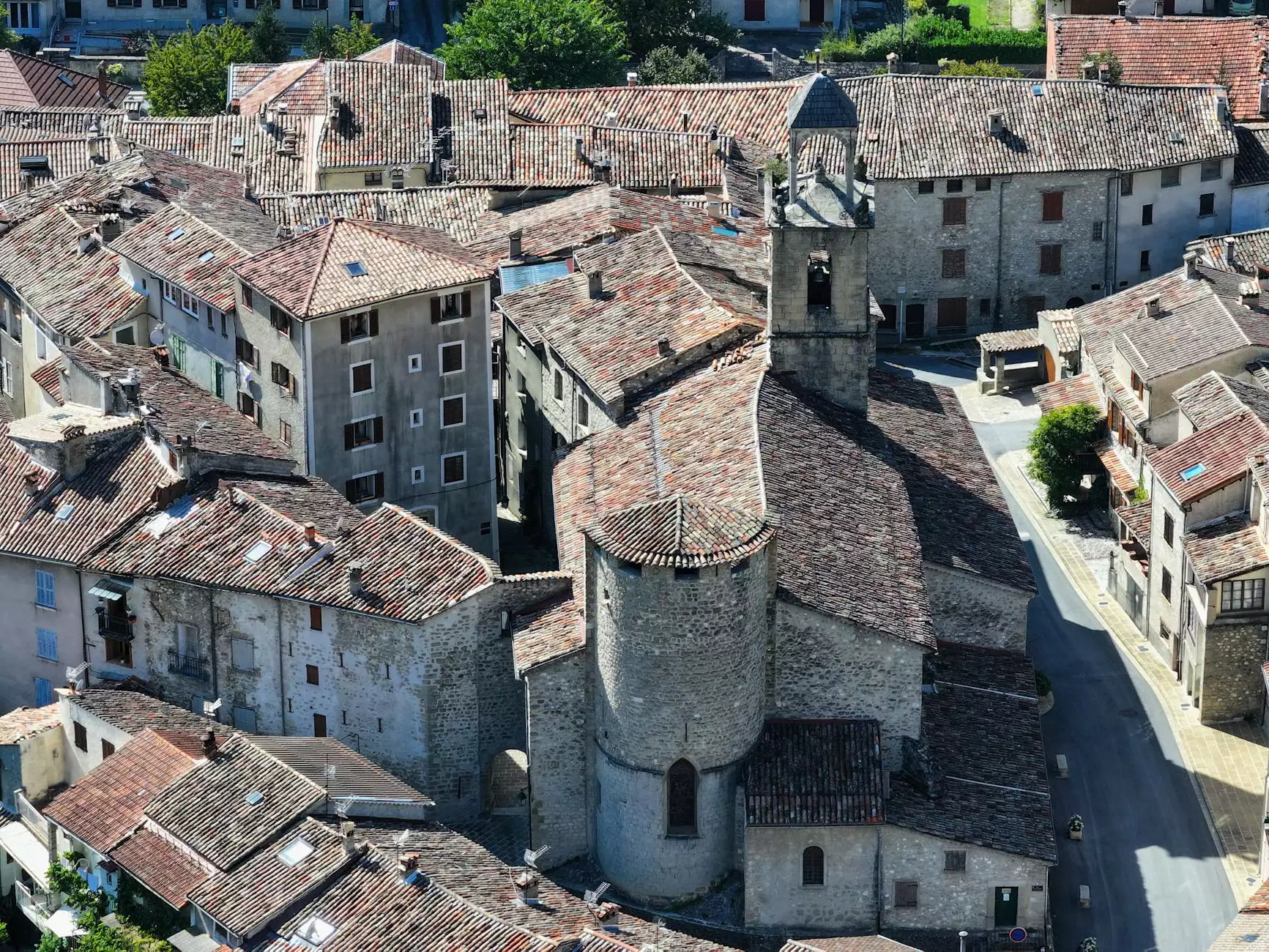
In the world of machine learning, the importance of data annotation cannot be overstated. As businesses strive to harness the power of artificial intelligence, the accuracy and quality of their machine learning models are often dependent on the quality of the data they use. This article delves into the critical role that data annotation plays in machine learning, showcasing its impact on businesses and how companies like Keymakr are paving the way.
What is Data Annotation?
Data annotation refers to the process of labeling data to make it usable for machine learning algorithms. This process involves identifying and tagging parts of a dataset so that machines can interpret the data accurately. Whether it is images, audio files, text, or video, data annotation transforms raw data into a structured format that helps machine learning models learn effectively.
Why Data Annotation is Crucial in Machine Learning
Without proper data annotation, machine learning models cannot function optimally. Here are several key reasons why data annotation is integral to machine learning success:
- Improves Model Accuracy: Annotated data provides the necessary context that allows models to make predictions more accurately.
- Enhances Training Processes: Well-annotated datasets reduce the time it takes for models to learn and refine their algorithms.
- Facilitates Better Insights: Insightful, annotated data can reveal trends and patterns that unlabelled data may mask.
- Supports Diverse Applications: Data annotation enables machine learning to be applied across various fields, including healthcare, finance, and retail.
The Types of Data Annotation
Data annotation encompasses several techniques, each tailored to specific types of data. Below are some of the prevalent types of data annotation:
1. Image Annotation
Image annotation involves labeling images by marking specific objects, features, or areas within an image. This is particularly important for computer vision tasks. Common image annotation types include:
- Bounding Box: Rectangular boxes are drawn around objects for detection.
- Semantic Segmentation: Each pixel is classified into different categories for segmentation.
- Landmark Annotation: Specific points in images are marked for facial recognition.
2. Text Annotation
Text annotation involves labeling textual data to help algorithms understand context and semantics. This type is crucial for natural language processing (NLP) and includes:
- Sentiment Analysis: Assessing sentiments behind text.
- Named Entity Recognition: Identifying entities like names, dates, and locations.
- Part-of-Speech Tagging: Tagging words based on their grammatical role.
3. Audio Annotation
Audio annotation involves labeling sound recordings to aid in speech recognition and audio analysis. Techniques include:
- Transcription: Converting spoken language into written text.
- Sound Event Detection: Identifying specific sounds or noises.
4. Video Annotation
Video annotation is essential for training models in applications like surveillance and autonomous driving. Common practices include:
- Frame-by-Frame Annotation: Labeling objects in each frame of a video for motion detection.
- Activity Recognition: Identifying activities and behaviors within videos.
Challenges in Data Annotation
While data annotation is beneficial, it also presents several challenges that businesses must navigate:
- Time-Consuming: Manual annotation can require substantial time and resources.
- Quality Control: Ensuring the accuracy of annotations can be difficult, and discrepancies can lead to poor model performance.
- Scalability: As datasets grow, maintaining efficiency in annotation processes becomes increasingly complex.
Data Annotation Best Practices
To maximize the benefits of data annotation, organizations should adopt the following best practices:
1. Leverage Specialized Tools
Using dedicated annotation tools can simplify the annotation process, reduce human error, and enhance productivity. Tools often incorporate features that standardize annotations and provide collaborative environments for teams.
2. Establish Clear Guidelines
Providing clear instructions and guidelines for annotators is crucial to maintain consistency. Training annotators effectively and developing a comprehensive annotation guideline document can dramatically improve quality.
3. Implement Quality Assurance Measures
Incorporating regular quality checks, peer reviews, and continuous feedback loops ensures annotations meet desired quality standards. This is vital for maintaining the integrity of the annotated datasets.
4. Utilize Automated Annotation
Combining automated tools with manual processes can significantly enhance efficiency. While automation can handle repetitive tasks, human annotators can focus on nuanced annotations that require context and judgment.
The Role of Keymakr in Data Annotation for Machine Learning
At Keymakr, we understand the pivotal role that data annotation plays in empowering businesses to succeed in the competitive landscape of machine learning. Our comprehensive data annotation services cater to diverse industries seeking accurate and efficient labeled datasets.
Why Choose Keymakr?
- Expert Team: Our annotators are skilled professionals who bring domain expertise, ensuring quality in every project.
- Advanced Technology: We leverage the latest tools and technologies to streamline the annotation process, maintaining efficiency without compromising quality.
- Customization: We understand that each project is unique, and we offer tailored annotation solutions to meet specific business needs.
- Scalability: Our services can efficiently scale to handle both small and large projects, making it easy for businesses to grow.
Future Trends in Data Annotation
As the demand for machine learning continues to rise, data annotation will evolve with notable trends shaping its future:
- Increased Automation: Advances in AI and machine learning may lead to more automated data annotation tools that require less human intervention.
- Real-Time Annotation: The need for speed will drive innovations in real-time annotation solutions, essential for applications like autonomous vehicles and live video analytics.
- Enhanced Collaboration: Future annotation tools will likely promote better collaboration among teams, improving multi-functional engagement and feedback integration.
Conclusion
In conclusion, data annotation machine learning is not just a process; it’s a critical element that can influence the success of AI initiatives across industries. By investing in quality data annotation, businesses can unlock the full potential of their machine learning models, enabling insightful and actionable outcomes. As a frontrunner in the field, Keymakr is committed to providing exceptional annotation services that empower businesses through high-quality data labeling solutions.